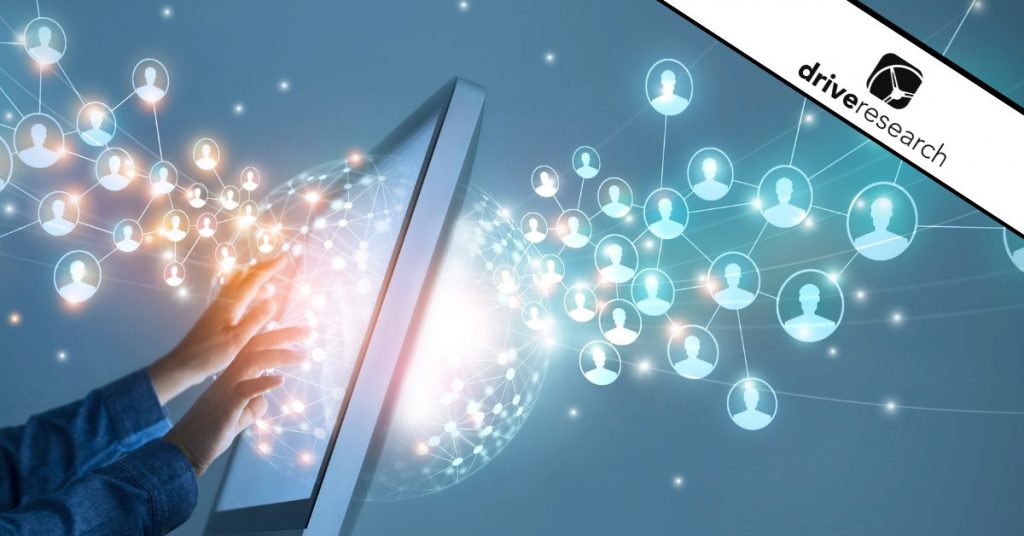
Multivariate analysis is like a powerful magnifying glass for data enthusiasts. It’s a set of techniques that helps us make sense of complex information by examining multiple variables at once.
Imagine you have a basket of fruits with various characteristics like weight, color, and sweetness. Multivariate analysis allows us to unravel how these traits relate to each other, revealing hidden patterns and insights.
In this blog post, we’ll dive deeper into multivariate analysis, exploring its types and providing examples to showcase how it uncovers valuable information for various objectives.
What is Multivariate Analysis?
Multivariate analysis is any type of statistical analysis that reviews more than one variable.
There is a lot of value in comparing the importance or impact of specific variables. For example, if you are launching a new product or service and the likelihood of purchasing is extremely low based on your survey results, you’ll want to know if this was caused by price, features, color, design, etc.
Using multivariate techniques in market research helps add clarity to this process and supports a data-driven strategic approach.
As I mentioned to a client the other day, “It helps quantify your gut feeling.”
Categories of Multivariate Analysis
1. Multiple Regression
This uses your long list of grid satisfaction ratings and works them into a model to predict which factor has the most impact on overall satisfaction or likelihood to purchase. It’s used often in forecasting.
It starts by asking your respondents their overall likelihood to purchase on a 1-10 scale followed by asking them the appeal of each of the following features (price, feature 1, feature 2, color, etc.) using the same 1-10 scale.
Using regression analysis you will discover the feature has the most significant impact on the likelihood of purchase.
Therefore it helps you understand priorities as to what features or benefits might cause a product failure and what needs improvement.
2. Conjoint Analysis
This is most closely associated with “trade-off.” It is asking…
- Would you rather have feature 1 or feature 2?
- Would you rather have color options or a lower price?
- Would you rather have feature 1 or color options?
In online survey research, conjoint analysis is a common form of trade-off analysis.
Just keep in mind, that if you have a long list of factors it may seem very repetitive to the survey taker, so look for ways to randomize lists across a series of respondents or limit your factors.
Long statistical models like this in surveys often increase the rate of drop-outs.
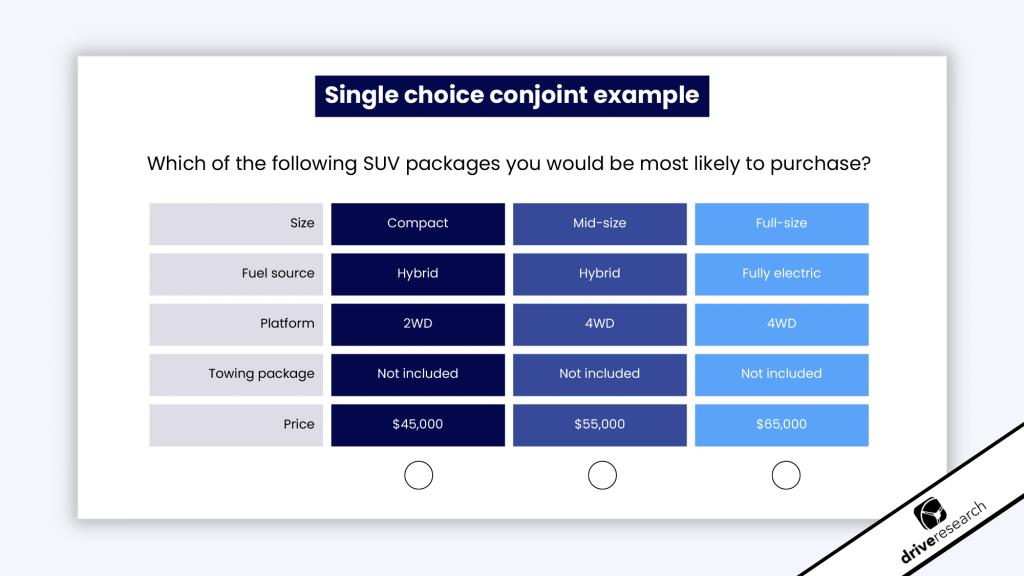
3. Discrete Choice Modeling (DCM)
This is similar to traditional trade-off conjoint but typically includes a series of variables. In this example of multivariate analysis, the survey designer creates a packaged concept and forces a choice. Think of it as almost a Package A or Package B question.
What is an Example of a Multivariate Analysis?
Imagine you work for a retail company that sells a wide range of products.
The company has gathered data on various customer attributes such as age, income, spending habits in different product categories (e.g., electronics, clothing, groceries), and their frequency of visits to the store.
The goal of the company is to understand its customer base better in order to tailor marketing strategies and product offerings more effectively. They want to identify distinct customer segments based on these attributes.
Here’s how multivariate analysis can be applied:
- Data collection: Conduct an online survey to collect data on customer attributes: age, income, spending habits, visit frequency, etc.
- Applying multivariate analysis: The analysis will identify new “principal components” that capture the most significant patterns of variation in the data.
- Customer segmentation: Using the scores on the retained principal components for each customer, you can now perform clustering techniques to group customers into market segments based on their similarities in terms of the principal components.
- Tailor marketing strategies for each customer segment: For example, if one segment is characterized by high spending on electronics, the company might target them with electronics promotions or advertisements.
In this example of multivariate analysis, the retail company is able to distill the complexity of customer data into a smaller set of meaningful components. This allows for a more focused and effective approach to customer segmentation and marketing strategies.
Contact Drive Research for Multivariate Analysis
Drive Research is a national market research company. Our team has a combined 80+ years of experience working with advanced survey analysis techniques such as multivariate analysis. If you are interested in working with our full-service online survey company, contact us today.